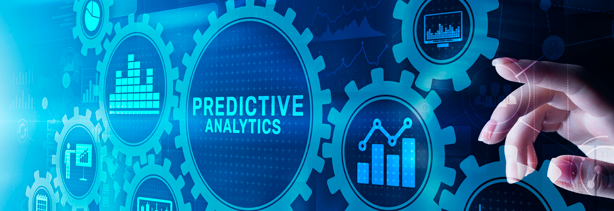
Globally, an increasing amount of data is produced every year. Organizations recognize the need to capture the value from this data but often fail to realize their ambitions. Business analytics is needed to analyze the data and generate valuable outputs. Business analytics can be divided into three categories: descriptive, predictive, and prescriptive analytics, each having their own enablers, issues and use cases. To become a highly mature data-driven organization, it is vital to lay a solid foundation that supports these distinct kinds of analytics.
Introduction
The global pandemic has affected our world in many ways. Everyone has experienced the dramatic effect that the pandemic has had on our personal lives. The effects have also been enormous for organizations around the world. Organizations have been forced to work remotely and have become even more dependent on technology. In a recent IDG study among approximately 2000 IT leaders, nearly 25% of the participants said that the pandemic has accelerated plans to improve their use of data analytics and intelligence ([IDG21]).
This clearly shows that the pandemic is an accelerator for a trend that has been ongoing for a while. Algorithms for predictive and prescriptive analytics have been around for decades, but only recently the amount of data is becoming enough to apply them in widely among industries, and the amount of data that is being generated continues to grow. After the online data explosion caused by COVID, the introduction of 5G and the rising numbers of operational IoT (Internet of things) devices will result in another rapid increase in the amounts of data that are produced every day. By 2030, 6G networks are expected to reach speeds of 1 terabyte per second and internet delivered via satellites will both accelerate the movement of data and reduce latency, opening the door for more advanced real-time analytical use cases. A growing number of organizations recognize this trend and are increasing their investment in analytics, aiming to improve their performance and gain a competitive advantage. In 2016, the global big data and business analytics market was valued at $130.1 billion. In 2020, this increased to more than $203 billion, at a compound annual growth rate of 11.7% ([Pres20]).
However, while most organizations acknowledge the need to become more data-driven, many of them fail to achieve the goals they are aiming for. 48% of organizations expect a significant return from investments in data & analytics within the next 3 years ([KPMG19a]). While 36% of organizations prioritize investments in data & analytics, only 25% of these initiatives have been successful ([KPMG19a]).
Figure 1. CEO’s view on D&A. [Click on the image for a larger image]
While most organizations are struggling to become more data-driven, there are examples that have beaten the odds and have shown that is possible to capture value from analytics and use their analytical insights to create a competitive advantage.
One of these examples is the Dutch online supermarket Picnic. Picnic built analytics into the core of their business. Decisions within organizations are increasingly and primarily based on data. Algorithms are used to calculate the exact need of personnel in warehouses and the fleet of delivery cars. The prices on the website are dynamic and based on competitor prices and demand. The product offering is based on a upvoting system and products are removed when they are not popular enough, to make room for more popular products. Picnic even uses data gathered from the delivery trucks to monitor safe driving Key Performance Indicators (KPIs), which have led to an almost 30% drop in harsh corners and speeding time. The rapid growth of Picnic is clearly fueled by being data-driven and making decisions based on analytics.
Descriptive, predictive, and prescriptive analytics
The need to utilize the full potential of the ever-increasing amounts of data has led to a remarkable evolution of technologies and techniques for storing, analyzing, and visualizing data. Due to innovations within business analytics, three distinct levels of analytics have emerged: descriptive, predictive, and prescriptive analytics.
Figure 2. Descriptive, diagnostic, predictive and prescriptive analytics. [Click on the image for a larger image]
Descriptive analytics
Descriptive analytics enable organizations to understand what happened in the past. Descriptive analytics is considered to be the “data summarization phase.” This kind of analytics summarizes raw data and answers the question “What has happened?” Within descriptive analytics, the subcategory diagnostic analytics tries to answer the question “Why did it happen?” Descriptive analytics makes use of simple periodic business reporting, ad-hoc reporting and OLAP techniques. The main objective of descriptive analytics is the identification of business problems and opportunities. Descriptive analytics is also often used for compliance and reporting purposes. Descriptive analytic outcomes tell you how much revenue you have generated last month and what the bestselling items were.
Predictive analytics
Predictive analytics tries to build sufficiently accurate models that predict the future by applying simulation techniques and machine learning algorithms to data. Organizations use predictive analytics to answer the questions “What will happen?” and “Why will it happen?”. Predictive analytics helps organizations to identify future risks and opportunities by extracting specific patterns from historical data. The volume of available data influences the quality of predictive analytics. When more data is available, models can be validated better, which can lead to more accurate predictions. Common methods and techniques that are used in predictive analytics are text/media/web mining, data mining, and forecasting methods. These methods are used to discover predictive and explanatory patterns (trends, affinities, associations, etc.) that represent inherent relationships between the input data and the output. The main objective of predictive analytics is to provide an accurate projection of future happenings and the reasoning as to why.
Amongst other things, predictive analytics can be used for cross-selling, prospect ranking, demand forecasting and customer retention. VodafoneZiggo is one of the organizations that successfully uses predictive analytics for customer retention. Every time there is a customer contact moment, data is gathered which tells them what they need, what they expect and whether they are satisfied. This information is used to improve their service provision ([Voda20]).
Prescriptive analytics
Prescriptive analytics prescribes the best decision option and illustrates the implications of each decision option to take advantage of the future. Prescriptive analytics incorporates the outputs generated by predictive analytics and uses optimization algorithms, artificial intelligence, and expert systems in a probabilistic context to present automated, adaptive, time-dependent, and optimal decisions. Prescriptive analytics can provide two kinds of output: decision support, which provides recommendations for actions, or automated decisions, in which case the algorithm executes the prescribed actions autonomously. Prescriptive analytics is the most advanced category of analytics and has the potential to offer the greatest intelligence and business value. How well the mathematical models incorporate a combination of structured and unstructured data and capture the impacts of decisions, determines the effectiveness of the prescriptions. Prescriptive analytics is a promising and emerging field of data analytics but is still very immature and not often adopted in organizations.
Possible use cases are dynamic pricing and production, marketing, or logistic optimization models. Ahrma is a Dutch technology firm that is revolutionizing the logistics industry by making use of IoT transponders. Ahrma produces pallets with IoT transponders that send data about locations, temperature, and weight. This data is summarized in a real-time dashboard which provides real-time insights in the logistic processes of Ahrma’s clients. Ahrma is taking its analytics to a new level. Together with KPMG, they are developing a prescriptive logistic optimization model that will benefit both Ahrma and its clients by decreasing costs and CO2 emissions and optimizing efficiency.
The building blocks of a solid analytics foundation
Each level of analytics requires a more mature and capable organization. This maturity should be in place for both the supply side, which is delivering insights, and the ‘consumer’ side, which is making business decisions. But organizations experience many barriers in their journey to improve their organizational (D&A) maturity.
Global KPMG research in 2019 has identified the most common issues that (financial) organizations experience, which is to a large extent representative for other large organizations that cope with many legacy applications and legacy data.
Figure 3. Biggest barriers to improving D&A maturity ([KPMG19b]). [Click on the image for a larger image]
Therefore, the issues mentioned in Figure 3 are seen in most sectors and large organizations. Most common issues are data availability/accessibility, data quality and generating value from the insights that are derived from the data.
To overcome these issues, organizations need to build a solid D&A foundation. This foundation consists of a set of building blocks that can be divided into three categories tangible resources (e.g. data, infrastructure, software), intangible resources (e.g. governance, culture, strategy) and human skills and knowledge (e.g. technical knowledge, analytical knowledge, data literacy). Organizations must focus on these tree components, before they can successfully perform descriptive, predictive and prescriptive analytics and generate business value from their efforts and investments.
Figure 4. Building block that form the D&A foundation. [Click on the image for a larger image]
Tangible resources
Data availability
For any form of analytics, data availability is vital. It is not only about the number of available data sources, but also about how well the data is documented and shared within the organization. Focusing on data lineage helps in having a better overview of the available data. Data lineage is the process of recording and visualizing how the data was transformed, what changed and why. Data catalogues are organized inventories of data assets in the organizations. Data catalogues give a better understanding of the data and increase operational efficiency. Organizations often have problems when it comes to the availability of data. They either don’t have enough data sources or there is a lot of data, which is unstructured and undocumented and therefore hard to use. To successfully make use of predictive and prescriptive analytics, organizations need larger amounts of data, data of lower granularity and data from more sources, both internally and externally. A growing number of organizations is turning to online marketplaces where they are able to sell and buy data.
Data marketplaces
With modern data platforms, organizations are able to process, store and analyze vast amounts of data. Over the last years, vendors of these type of platforms started to integrate data marketplaces in their service offerings. A key example of this is Snowflake, a cloud data platform vendor who made the data marketplace a key pillar of their service offering. Using their platform, organizations are able to easily integrate external datasets from vendors or produce data sets for customers. For example, organizations are able to buy online behavioral data in order to better understand, expand and validate consumer behavior for targeting and analytics in order to achieve better conversion rates on their online platforms.
The growth of data marketplaces is an inevitable result of the IoT revolution. As physical assets such as ships, factories, vehicles, farms, and buildings become equipped with smart connected technology, their digital “twins” produce constant streams of valuable data points. These data streams surge across silos and carry value across organizations. Data marketplaces emerge as a means to exchange data, monetize data streams, and provide the basis of new “smart” business models ([IOTA19]).
Data quality
Data quality determines how well analytics can be performed. Inferior quality data leads to inaccurate analytics and insights. Data quality is a key enabler for most use cases. See for examples where improving data quality was a key enabler in the insurance or food retail industry ([Lust18], [Rijs17]). To be of high quality, data must be consistent and unambiguous. To achieve this, organizations should have centrally documented data definitions and business rules. Most of the leaders in this area have implemented Data Catalogues in order explicitly document these definitions and rules. By combining these Data Catalogues with strong Data governance and Master data management, these organizations see a significant increase in efficiency of their development teams and have shorter lead times for their data projects.
Many organizations are having issues with data quality. Reasons for data quality issues: non-compatible legacy systems, separation of data in silos, unskilled employees, and missing documentation of the data. Even the most mature organizations are not fully satisfied with their data quality. The more data-driven an organization becomes, the bigger the impact of data quality on the organization and its decision-making processes.
There are two reasons for this increasing importance of data quality: firstly, data-driven organizations base more decisions on their data. When the data is flawed, more decisions are impacted. Secondly, Employees in mature organizations have less doubt about the quality of the data. They are used to base decisions on insights from their data and are less likely to question the data quality.
IT infrastructure
Descriptive analytics requires a modern data warehouse (or data hubs/lakes) that can store data from different siloed systems in a structured way. An outdated or siloed infrastructure leads to issues with data availability and quality. When a central infrastructure is lacking, organizations try to upload data from different silos into a single BI tool. However, the different silos often contain contradictions, making it hard to define the truth. Infrastructures like data lakes can work with outdated legacy systems and enable the organization to create a common reality. Predictive and prescriptive analytics require not only BI tooling but often multiple data science tools. Organizations need a modern data platform that can handle multiple data sources and supports different D&A toolsets. Most leading organizations are utilizing cloud-based platforms because of their scalability, agility, and cost structure.
Analytical software and tools
Ideally, organizations have standardized tools for every category of analytics (and visualization thereof) that fit the organizational requirements. Having a standardized set of tools helps the organization in multiple ways. There will be less maintenance, fewer license fees, and less needed knowledge. Business users will be more used to the user interface; they are therefore more likely to use the tools and base their decisions on the provided insights.
Low mature organizations often do not have standardized toolsets and either do not have the right tool for their analytics purposes or every department has its own tool. Too many different tools are non-desirable because of the extra costs and maintenance but also employees are less likely to use insights from tools that they are not familiar with. This is especially the case for data visualization tools since the output from these tools is used broadly throughout the organization by less experienced business users. Mature organizations have set up a standardized toolset for descriptive, predictive, and prescriptive analytics. Modern cloud-based tools hardly have any limitations in their capacity to perform all types of analysis. However, most modern tech ‘unicorns’ go even further; their tooling is fully embedded into the day-to-day business applications and enables data-driven business processes and decision-making.
Intangible resources
D&A strategy
A well-developed long-term D&A strategy guides an organization in becoming increasingly data driven. The focus of the D&A strategy is largely dependent on the maturity level of an organization. The strategic goals and the KPIs must be aligned with the analytic capabilities. When descriptive analytics is still something an organization struggles with, then the focus should be on upgrading legacy systems, compatibility, and standardization. Only when descriptive capabilities are embedded in the organization, it is time to start looking at predictive and prescriptive analytics. When developing the D&A strategy, a long-term focus should be kept in mind. Management is too often primarily concerned with short-term results (ROI) instead of focusing on long-term objectives and so-called decision-driven data analytics (see also [Lang21]). Funding is a bigger issue for predictive and prescriptive analytics since management is less familiar with these kinds of analytics. However, using more advanced analytics takes time before it starts generating value.
The most data-driven organizations are already using descriptive, predictive, and prescriptive analytics throughout the business. Traditionally, reporting was often driven by finance and control departments. Predictive and prescriptive analytics have enabled many more use cases that allow for value creation throughout the organization. Analytical use cases can now be found in finance, marketing, supply chain, HR, audit, and IT departments. A recent development is the focus on automation of processes. In the most advanced cases, this means that outcomes from prescriptive analytics trigger automated processes that are performed by robots.
D&A Target Operating Model
The setup of the organizational structure, including spans of control and layers of management, plays a critical role in scaling standardized analytics throughout the organization. The governance structure defines the management, roles, and oversight of an organization. It shows the decision-making, communication and management mechanisms concerning analytics. First, an organization should decide whether it wants to have decentralized analytics units throughout the organization or if it prefers a centralized analytics unit. Both options can work as long as there is an efficient governance put in place.
Complex governance is an issue that hinders the value generation of analytics. Hierarchical and multi-layered governance slows down the entire process of adopting innovative technologies for analytics. Mature data-driven organizations keep governance concerning innovative initiatives as simple as possible. These organizations have cross-functional independent product teams that are integrated into the business. These teams have a large amount of autonomy to decide how they want to operate. A set of best practice guidelines is provided, supplemented by strict rules concerning data privacy and security, based on security/privacy policies and data classification. Such a fundament ensures flexibility and adoption of new and innovative techniques. For sensitive personal data, organizations might have to make use of the Data Protection Impact Assessment (DPIA). This is an instrument for mapping the privacy risks of data processing in advance and then take measures to reduce the risks, the GDPR mandates a DPIA in case of potential high privacy risks.
Data-driven culture
A data-driven culture stimulates the use of analytics throughout the organization and increases the acceptability of the outputs that analytics generates. In data-driven organizations, the norm is that arguments and decisions must be based on insights generated from data. Developing a data-driven culture seems to be especially important when it comes to predictive and prescriptive analytics. When there are doubts about the quality of the outcomes, employees prefer to base their actions on their feelings. More advanced analytics means that there is less input needed from employees in the decision-making processes. One of the issues with predictive and prescriptive analytics is the ‘black box.’ When stakeholders do not know how algorithms or data analytics work, they are less likely to use the outputs for their decision-making ([Praa19], [Verh18]). Therefore, organizations should prefer to have less accurate but more explainable predictive and prescriptive algorithms. Another key factor within the culture is the willingness to change and innovate. Employees have to be willing to change their habits, such as abjure their Excel addiction, and adopt innovative technologies. Creating a data-driven culture should be a priority in the broader corporate strategy.
White boxing
Machine learning has immense potential for improving products and processes. But models usually do not explain their predictions which is a barrier to the adoption of this type of analytics ([Moln21]). Next to a barrier of adoption, these black box algorithms also can become biased or unethical.
Organizations are trying to use an innovative approach, named White Box AI, and focuses on models which can be interpreted by humans. These white-box models can explain how they behave, how they produce predictions and what the influencing variables are. There are two key elements that make a model white box: features must be understandable, and the ML process must be transparent ([Scif20]).
As increasingly advanced analytics is embedded into business processes, there is a growing need for these explainable models. Organizations need to ensure that their algorithms are complying with GDPR and within their ethical boundaries. For example, the Dutch Tax Office was targeting minorities using risk models based upon features which were later considered to be illegitimate to use for this purpose ([AP20]).
Human skills and knowledge
Skills analytics team
A skilled analytics team enables an organization to realize its ambitions. Depending on the maturity of the organization, the analytics team should have data engineers, data scientists, data architects, visualization specialists and most critically: translators who form the bridge between the analytics department and the business. When a D&A infrastructure and operating model are lacking and the organization is not yet adequately performing descriptive analytics, most organizations should focus on hiring all-round ETL, database and BI developers. The more technical data analytics roles can be contracted (filled in by freelancers) or outsourced (e.g. BI-as-a-Service), the more functional roles should preferably be established internally. Organizations that have to deal with many legacy systems must mitigate the risk of key person dependencies. Too often vital knowledge is only known by a few experienced employees. There is always a risk that knowledge will be lost when it is not well embedded in the organization. Many organizations experience the high demand concerning all data-related skills and find it difficult to attract the right people and are therefore forced to hire freelancers, in which case the risk of key person dependency should also be mitigated. Sometimes it is a better option to train and develop internal resources into the required profiles. D&A teams can benefit from context knowledge and employees are possibly more loyal because of development opportunities. Only when the foundations are laid and the organization is ready for larger amounts of real-time data, it becomes relevant to start hiring data engineers and data scientists. There are many examples where organizations rushed into hiring data scientists while the foundations were still missing. These data scientists were either forced to leave because there were no use cases, or they had to perform tasks that could have been done by less expensive data engineers.
Data literacy
Even when organizations can perform accurate and useful predictive and prescriptive analytics, they are often still not generating business value from these techniques. This is not due to technical limitations, as development and innovation in the field of data analysis are unfolding rapidly. The lack of business value is often caused by the gap between data professionals and business users. Users have a key role in understanding and analyzing the outcomes of data products and to turn their analysis into business insights, actions, and value. The gap between data and business professionals, often defined as a lack of data literacy throughout the organization, can be alleviated by further educating the organization on data concepts, cultural change programs and data-driven employee reward programs ([Goed18]). All hierarchical levels in the organization must have at least a basic understanding of data concepts and must be able to understand and engage with the data that suits their role. Only when the employees understand the data concepts, it becomes possible to make the right decisions based on the created insights. Another way to bridge the gap is with agile multidisciplinary teams. This iterative methodology invokes alignment between the demand and supply side of analytics, leading to shorter throughput times for novel solutions, more cross-functional knowledge and better management of focus and priorities.
Conclusion
Organizations aiming to generate value from descriptive, predictive, and prescriptive analytics must use a comprehensive approach to data analytics. Their endeavor is as strong as the weakest link in the set of required tangible resources (data/IT infrastructure), intangible resources (governance, culture, strategy) and human skills and knowledge (analytical competencies, data literacy). Even when have the best-in-class tools and infrastructure in place, without a strong data culture and appropriate data quality, outcomes of analytics will be almost worthless. Therefore, mature data-driven organizations have strengthened all these components.
This comprehensive approach is not limited to the supply side of data and analytics. The outcomes of these insights should be embedded in the business and its processes. Managers and employees must use the output from analytics and base their decisions on this output.
A truly data-driven organization is able to deliver and consume analytics, fully capturing the value of their data.
References
[AP20] Autoriteit Persoonsgegevens (2020, July 17). Werkwijze Belastingdienst in strijd met de wet en discriminerend [Dutch]. Retrieved from: https://autoriteitpersoonsgegevens.nl/nl/nieuws/werkwijze-belastingdienst-strijd-met-de-wet-en-discriminerend
[Goed18] Goedhart, B., Lambers, E.E., & Madlener, J.J. (2018). How to become data literate and support a data-driven culture. Compact 2018/4. Retrieved from: https://www.compact.nl/articles/how-to-become-data-literate-and-support-a-data-driven-culture/
[IDG21] IDG/CIO Magazine (2021, March). No Turning Back: How the Pandemic Has Reshaped Digital Business Agendas. Retrieved from: https://inthecloud.withgoogle.com/it-leaders-research-21/overview-dl-cd.html
[IOTA20] IOTA Foundation (2019, February 25). Onboard the Data Marketplace. Part 1: IOTA Data Marketplace – Update [Blog]. Retrieved from: https://blog.iota.org/part-1-iota-data-marketplace-update-5f6a8ce96d05/
[KPMG19a] KPMG (2019). Agile or irrelevant: Redefining resilience: 2019 Global CEO Outlook. Retrieved from: https://home.kpmg/xx/en/home/campaigns/2019/05/global-ceo-outlook-2019.html
[KPMG19b] KPMG (2019). Future Ready Finance Global Survey 2019. Retrieved from: https://home.kpmg/xx/en/home/insights/2019/09/future-ready-finance-global-survey-2019.html
[Lang21] Langhe, B. de, & Puntoni, S. (2021, December 7). Leading With Decision-Driven Data Analytics. Sloan Management Review, Spring. Retrieved from: https://sloanreview.mit.edu/article/leading-with-decision-driven-data-analytics/ and https://sloanreview.mit.edu/video/understanding-decision-driven-analytics/ (video)
[Lust18] Lustgraaf, M. van de, Sloots, G.I., Rentenaar, B. Voorhout, M.A., & Koot, W. (2018). Is data the new oil for insurers like VIVAT? Harvesting the value of data using a digital strategy. Compact 2018/4. Retrieved from: https://www.compact.nl/articles/is-data-the-new-oil-for-insurers-like-vivat/
[Moln21] Molnar, C. (2021). Interpretable Machine Learning: A Guide for Making Black Box Models Explainable. Retrieved from: https://christophm.github.io/interpretable-ml-book/
[Praa19] Praat, F. van, & Smits, R. (2019). Trusting algorithms: governance by utilizing the power of peer reviews. Compact 2019/4. Retrieved from: https://www.compact.nl/articles/trusting-algorithms-governance-by-utilizing-the-power-of-peer-reviews
[Pres20] Press, G. (2020, January 6). 6 Predictions About Data In 2020 And The Coming Decade. Forbes. Retrieved from: https://www.forbes.com/sites/gilpress/2020/01/06/6-predictions-about-data-in-2020-and-the-coming-decade/?sh=661e54464fc3
[Rijs17] Rijswijk, R. van, Ham, R.F. van der, & Swartjes, S. (2017). Data Quality GS: The importance of data quality in the food industry. Compact 2017/1. Retrieved from: https://www.compact.nl/articles/data-quality-gs1/
[Scif20] Sciforce (2020, January 31). Introduction to the White-Box AI: the Concept of Interpretability. Retrieved from: https://medium.com/sciforce/introduction-to-the-white-box-ai-the-concept-of-interpretability-5a31e1058611
[Verh18] Verhoeven, R.S., Voorhout, M.A., & Ham, R.F. van der (2018). Trusted analytics is more than trust in algorithms and data quality. Compact 2018/3. Retrieved from: https://www.compact.nl/articles/trusted-analytics-is-more-than-trust-in-algorithms-and-data-quality
[Voda20] VodafoneZiggo (2020). “Data is the source of success and customer value” [Interview Aziz Mohammadi, director Advanced Analytics]. Connect Magazine. Retrieved from: https://www.vodafoneziggo.nl/magazine/en/big-data/data-de-bron-voor-succes-en-klantwaarde/